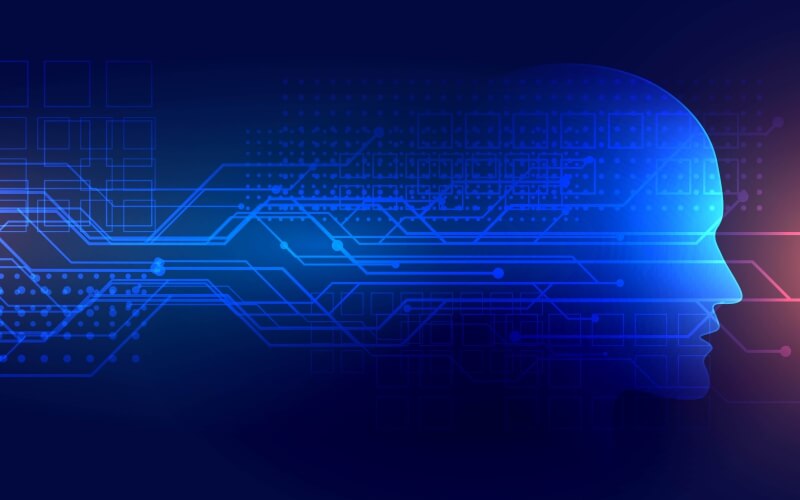
Machine learning, artificial intelligence, and deep learning have been gaining popularity for a long time. They are useful technologies that have been transforming many industries, such as finance and retail. However, healthcare is one of the industries where these technologies are being used the most. This is because healthcare is important, and medical professionals are always looking for new ways to use these technologies to provide better results.
Deep learning can have a huge impact on healthcare. It collects a lot of data, including data from patients, health records, and medical reports. Then it uses neural networks to figure out the best way to help people. Deep learning has already made a big impact in healthcare by helping with patient monitoring and diagnosis. The global deep learning market is expected to grow a lot in the next decade.
Deep learning technology is a way to make sure that your computer can learn things that it couldn't learn before. It does this by using a special kind of network called a neural network. This lets the computer work harder and get better results. Deep learning technology is useful because it can help you understand data in several layers.
Deep learning can help healthcare professionals find hidden opportunities and trends in data. This can help them provide better treatment to their patients. Deep learning models are good at completing classification tasks, such as detecting subtle abnormalities in medical image data, grouping patients with similar characteristics into risk-based cohorts, or highlighting relationships between symptoms and outcomes within vast quantities of unstructured data. Here are some of the most remarkable deep learning applications in healthcare.
Medical imaging and diagnostics – Deep learning techniques help us diagnose medical problems. They can interpret MRI scans, X-rays, and CT scans. This helps us find any risks or irregularities in the images.
Drug discovery – Deep learning is very important in discovering drug combinations. It was used to help develop drugs and vaccines during the pandemic. Deep learning algorithms can handle a lot of different data, like clinical, genomic, and population data. This lets researchers do things like molecular modelling and predictive analytics faster.
Personalized medication – Healthcare providers can use deep learning models to understand patients better. This helps them give individualized treatment. These technologies also allow for the detection of different treatment options for different people.
Clinical trials made simple – Machine learning and deep learning can be used to predict who might be a good candidate for a clinical trial. This will help scientists find people from many different data points. Deep learning will also allow trials to be monitored with less human interaction and fewer mistakes.
Some other examples of how deep learning is being used in the medical industry are fraud detection, improved health records and patient monitoring, and Alzheimer's disease detection.
Scientists and researchers are still experimenting with advanced applications in the healthcare sector. This helps to improve healthcare offerings. Recently, a scientist spoke about how deep learning can help to diagnose and monitor brain disorders. She said that brain abnormalities and neurodegenerative diseases are some of the most costly diseases in terms of human suffering and money.
Neuroimaging techniques like MRI, functional MRI, PET, and SPECT can help diagnose brain disorders. But these images are not usually used well to analyze them. Deatsch and her team created a new deep learning model that can tell the difference between brain scans of Alzheimer's patients and normal people. They also looked at how different things affected the model's performance.
Another study that is attracting attention is one that used deep learning to diagnose acute ischemic stroke. The study was conducted by MD, the director of the University of Maryland Medical Intelligent Imaging Center, and colleagues from Johns Hopkins University's Computer Science team. They built a high-performing system that can detect CTA of the head and LVO. The researchers found that using preprocessed images helped 2D CNNs perform much better, with AUCs more than 0.95. However, 3D CNNs did not perform as well, with AUCs ranging from 0.8 to 0.81. Dr. Yi believes that if the team's discovery is refined and clinically verified, it could help clinical radiologists in stroke care.
Technology has come a long way in the healthcare industry. There are now deep learning applications that can help with diagnostics. There are also other studies that show promise for better clinical improvements.